August 2021 • Issue 4
We deliver advanced neural network solutions for industrial clients, helping them improve their products and processes.
AI in Industrial Applications
Business benefits in real deployments. The following customer success stories offer a sense of how AI can strengthen a range of industrial applications.
Interview with Olga Kaiser, Research Scientist at NNAISENSE
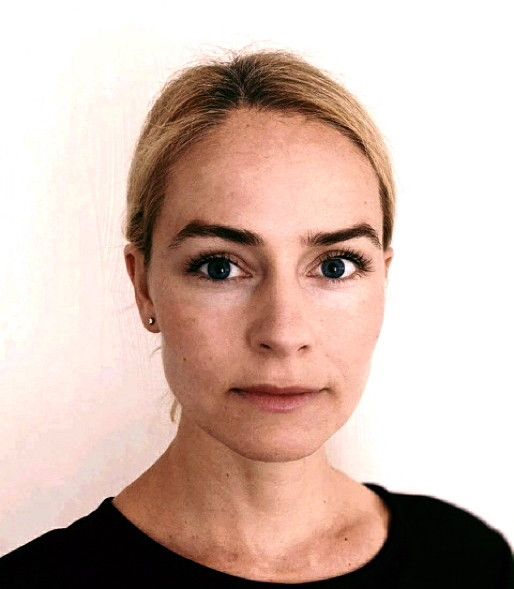
Hi, Olga. Great to have you answer my questions. What is your educational background and how long have you been at with NNAISENSE?
Hi there, I studied Mathematics and Physics with a focus on fluid dynamical systems. In my PhD studies, I worked on advanced methodologies for the analysis of spatiotemporal data with a focus on extreme meteorological events. I joined NNAISENSE three years ago
You have a great deal of experience running AI projects for our clients. What is most important when it comes to getting a project done to the client’s satisfaction?
It’s is not enough to get state-of-the-art results in plain numbers. Rather, the most important factor contributing to the success of a project is the collaboration with the client, and the desire to understand deeply the problem to be solved. Let me elaborate on this. In the beginning of every project, it’s very important to formulate the task, to set the objectives and to define the metrics—both mathematical and business— used for the evaluation of the solution.
These steps require a deep understanding of the technological processes. They are necessary because the delivered solution must fit into the customer’s production pipeline, taking into account the conditions when making decisions and the order of actions performed by the engineers responsible for controlling the technological process. This is very often an iterative process, and decisions made here will influence the way the models are designed and trained.
Furthermore, one of the most important steps in any project is data preprocessing and feature engineering. The latter includes extracting factors that are hidden in the data such as physical/chemical dependencies which can be identified only by incorporating domain-specific knowledge. This knowledge, it’s worth noting, can be obtained by reviewing the relevant literature and a close collaboration with the client.
Another important aspect involves integrating the AI solution into production. The deployed solutions needs to be reliable, sufficiently transparent, and, of course, explainable.
We talk a lot about AI-powered digital twins. What is your definition of a digital twin (DT), and how important is it in industrial use cases?
A digital twin is a model of a process under investigation that reliably describes the main characteristics of the underlying dynamics. This model is either based on first-principles or, more generally, on statistical concepts incorporating advances in AI such as deep learning that allow complex non-linear dynamics to be learned directly from sensor data. These data-based DTs not only enable improved forecasting of process dynamics, they also do it much faster due to the massively parallel processing of neural networks. It provides predictive diagnosis and can be supportive in making decisions and providing recommendations.
The importance of DTs is clear: they can enable faster, safer, and more efficient production. Simultaneously, we can maximize the production quality, all while reducing energy consumption and equipment wear (and therefore, reducing our overall environmental impact).
” the most important factor contributing to the success of a project is the collaboration with the client, and the desire to understand deeply the problem to be solved. “
One of our clients is Schott AG where we built a user assistance system (UAS). How can this type of DT be used in other industrial processing use cases in totally different industries like metal, chemical, food processing etc.?
Yes, this is a very good example of a DT. Together with Schott we designed a DT that can describe the underlying dynamics of the very complicated—and still not well understood—glass-melting process. The resulting model accounts for domain-specific characteristics like non-stationarity and multi-scale behavior (present in most industrial use cases).
For every single industrial use case, the DT needs to be customized. A successful solution requires the incorporation of relevant domain knowledge and, of course, specific customer requirements. However, during this project we gained significant expertise in designing DTs for complex processes where the amount of data is very limited, and the requirements include exposing the underlying causal structures.
You mentioned several times the importance of interpretability where DTs are concerned. Why is this important?
One of the main requirements for AI solutions in industrial applications is that they be trustworthy. The client expects that some basis for the recommendations made by the model be provided.
In the case of models based of first-principles, this requirement is fulfilled, since the analytic form is known a priori, but when it comes to AI it’s important to distinguish between “interpretability”and “explainability”. Explainability refers to making sense out of a black box model once it’s trained (e.g. a neural network), whereas interpretability refers to building models in which the inner workings are understandable by design (i.e. not a black box). The degree to which either of these can be provided depends on the choice of model class for a given application. For some industrial use cases interpretability may take precedence over precision. For others raw performance might be the driving factor, and powerful models such as deep network are used which are only amenable to explanation.
Another important requirement for industrial projects is the inference of cause-effect relationships. Causality Learning is a very active field in DL/ML, and is discussed at length by the pioneers of deep learning. Specifically, Causality Learning aims at learning the causal variables and understanding their effects on the environment, rather than inferring statistical correlations in the data. Incorporation of “structural causal models” and the inference of “causal mechanisms” is crucial for a DT, improving its overall performance in terms of stability, reliability, and of course the quality of its recommendations for the decision making processes.
As you are well aware, unfortunately, the field of AI (and Tech in general) is still overwhelmingly male-dominated. What measures do you think should to be taken to remedy the current situation in the long term?
Yes, according to recent statistics, only around 20% of the workforce in AI is female, and 27% in STEM. Compared to the numbers in 1970 when only around 8% were female in STEM, we are getting better, but progress has been very very slow. We still need to reduce gender stereotypes that are already present in kindergarten.
Inspiring girls with female role models and provide more learning opportunities like tech summer-camps is key. At this point, I’m not a supporter of girls-only training, but rather a supporter of a gender-free STEM environment. It is also very important to keep promoting public awareness to parents about the necessity to motivate and support the growth mindset of girls and to encourage their eduction in math and science.
Recent News – AI in Industrial Processes
IoT, Industry 4.0, Manufacturing, Industrial Processes
Stanford HAI has released its 2021 AI Index
The Index is an independent program developed by an interdisciplinary team at HAI, in partnership with academic, industrial, and government entities. It takes a comprehensive look at AI, including the progress of AI year on year – analyzing and distilling patterns involving the impact of AI on everything from national economies, to job growth, diversity, and research. Some of the 2021 AI Index findings:
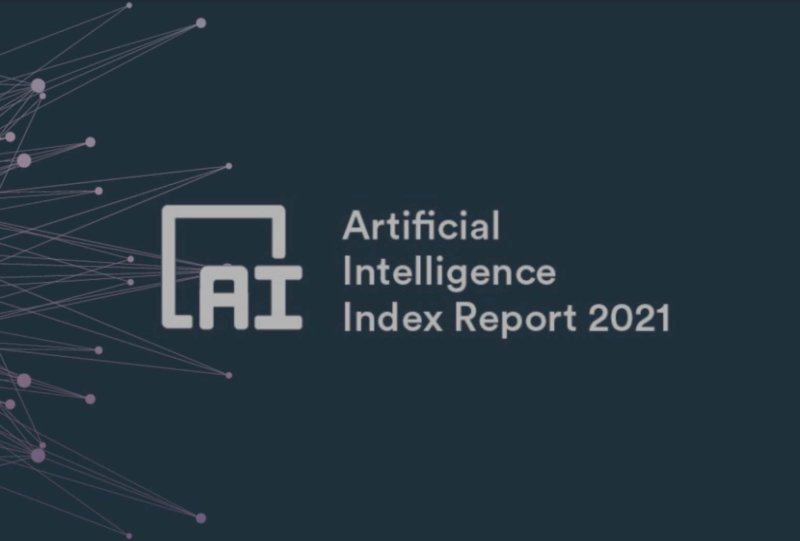
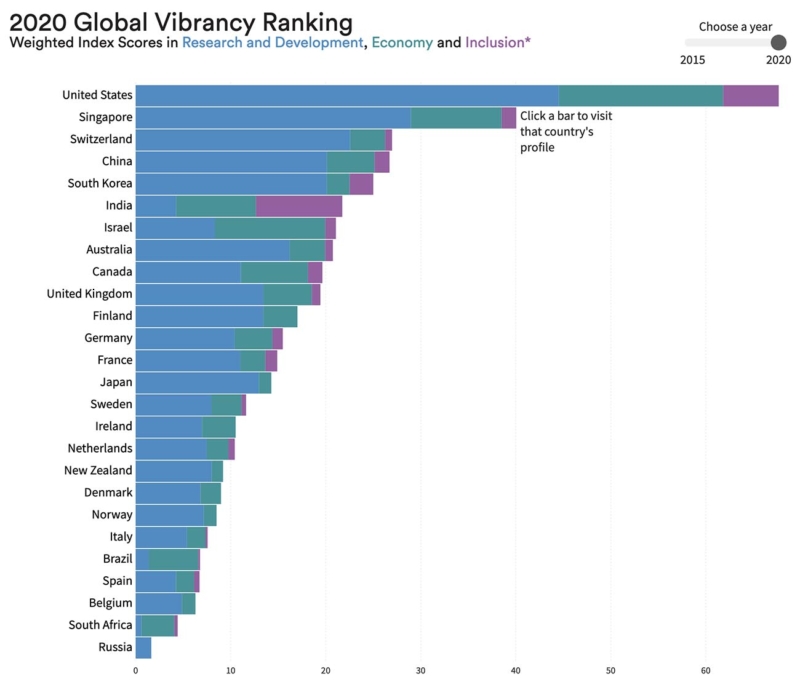
The 2020 Ranking cites the U.S., Singapore, and Switzerland as leading countries, with China in fourth place. It is interesting to see that there isn’t a single European country in the top 10.
Read Full Report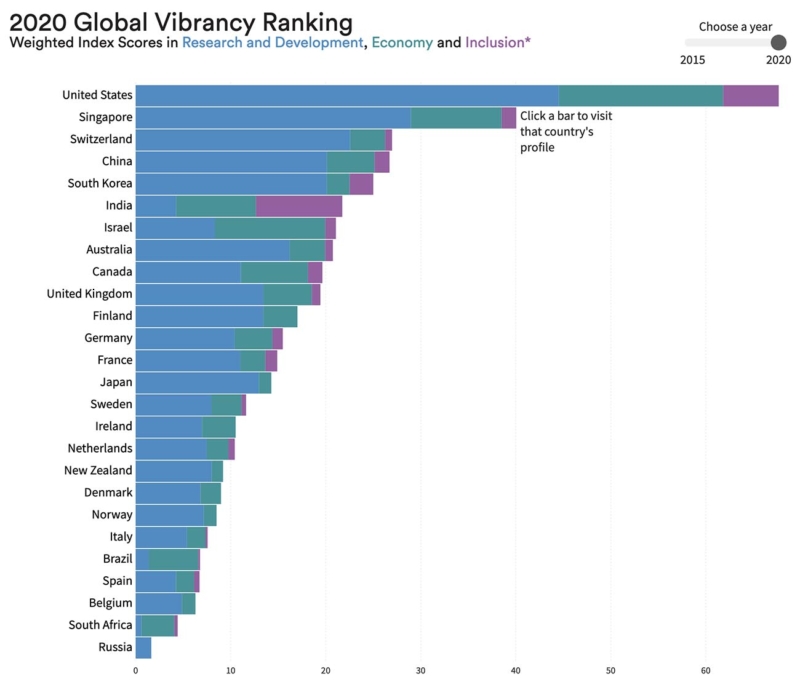
Nnaisense News
NNAISENSE Named a Gartner Cool Vendor
NNAISENSE was granted the prestigious Gartner AI Cool Vendor label in the AI Core Technology category. As the leading IT enterprise analyst, Gartner’s word means a great deal to us—and we are very proud that after six months of extensive discussion with Gartner AI experts, we received this honor.
Gartner List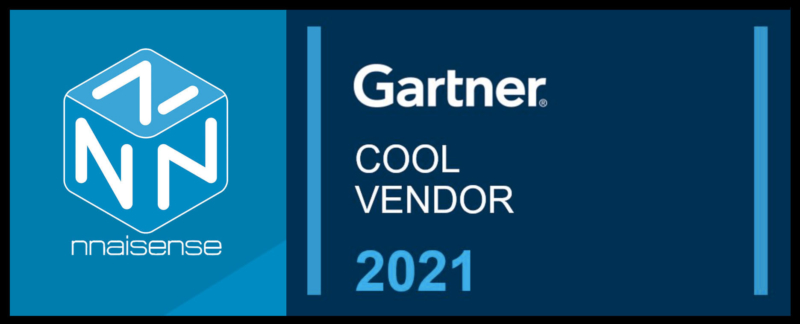
Announcing the Launch of
AI Celebrity Interview Series
Every month moving forward, we will conduct an interview with a well-known AI celebrity—asking them questions about their perspectives and experiences surrounding Industrial AI.
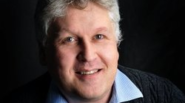
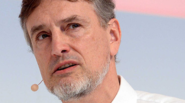
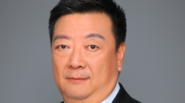
Dr. Pei Wang
Associate Professor at Temple University.
Department of Computer and Information Science
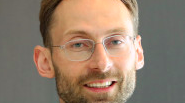
Introducing Industrial AI Academy
NNAISENSE will launch the first-ever Industrial AI Academy. Participants can enroll in one of two tracks (management or technical expert) and obtain a certificate upon completion of the program.
To stay informed with the latest news and updates, please sign on to the International Industrial AI Application Summit. From there, you will receive more information on what the program will be and how to enroll.
Interested in learning more about our Industrial AI Academy? Please contact Ralf Haller
Recent Press
- Machine Design
AI-Driven Digital Twins and the Future of Smart Manufacturing
(July 23, 2021)
- Silicon Canals
Europe is losing the AI wars. Is it doing enough to get back in the game?
(July 13, 2021)
- The Verdict
Digital twins in energy are powerful and putting out fires
(July 7, 2021)
- Today’s Motor Vehicles
The next wave of AI-powered networks
(June 17, 2021)
- VentureBeat
Password authentication is a mess. Here’s a system to replace it
(May 31, 2021)
- FAZ.net
Every 30 years Artificial Intelligences get a million times bigger
(May 17, 2021)
- Forbes
Machinery that (does not) think (in German)
(April 19, 2021)
- Hannover Messe Keynote
3 Real-world Industrial AI Use Cases
(April 13, 2021)