April 2021 • Issue 3
NNAISENSE delivers advanced neural network solutions for industrial clients that improve how their processes and products work.
AI in Industrial Applications
Business benefits in real deployments, customer success stories
Interview with Jonathan Masci, Co-Founder and Director of Deep Learning
Hello Jonathan, in the many AI projects that you and your team have done over the years, which one was the most complex and rewarding?
Ciao Ralf, I must say that every project is special and rewarding in different ways, so this is a very hard choice for me. However, the User Assistance System that we developed for SCHOTT AG to improve their specialty glass production is definitely a milestone for industrial AI. From raw historical sensor readings we were able to build a 3rd generation digital twin (3DT) of the glass melting process. The 3DT was then embedded into a deep analytics engine where the expert operator, always in synergy with our AI, can gain further insights into the process and confirm reasons for historical process deviations for trust building. And, as the cherry on top, recommendations are provided to improve the desired business KPI by a targeted 10%. Why was this difficult? These processes are highly non-linear and exhibit non-stationary behavior: wear and tear and unobservable factors change the observed dynamics of the system throughout the course of its operation. In addition, due to the perfected production processes built over many years, historical data is repetitive and contains very little information about undesirable process dynamics. Interacting with the process to collect more data is costly and more importantly risky, which means our AI system had to learn from very few samples.
How would you compare your experiences in first projects that you did when you started back at IDSIA 10 years ago with the ones you do now with NNAISENSE?
I was always passionate about project management, and I surely did my best since early days at IDSIA. However, to be completely frank, when you are in academia, publishing and raising your scientific profile are key motivators. Simply put, you’re in love with your ideas and completely disconnected (disinterested) from the business aspect that is behind a specific industrial research area. At NNAISENSE, we are driven by technology that works, is actionable, and provides our partners true benefits. The key is to understand where AI is essential, and that it is a cog in a very complex machine buy tiktok followers. Our role is to design technology that fits. In an academic setting, the research is its own end, whereas for us industrial AI is instead an enabler for better products and processes. Just having the technology adds little value, integrating it so that it can actually benefit your process is what really matters.
What type of challenges do you see in Industrial AI projects, and how do they differ from say an NLP-type project where one can use cloud-based off-she-shelf solutions?
The progress made in NLP in the recent years is unprecedented. It could actually generate the rest of this interview and you would not even notice, and perhaps it did. Behind this development are massive datasets and clever models like GPT-3 from OpenAI, but most importantly a shared semantics among all English written text. This makes it easy to standardize models and serve them on the cloud. The same cannot be said of industrial processes. Each is different, and even those that are designed to be identical differ due to the impossibility of having identical physical realizations of the same process. This is the reason why no off-the-shelf solution exists, yet. More importantly, there are no massive datasets, and possibly there never will be in many applications due to the process being slow and/or dangerous to operate in certain regimes. It would simply take too much time and cost too much. At NNAISENSE, we are building tools to run industrial AI projects as conventional NLP projects are run now: Predictable results and smooth delivery with little risk involved. Our goal is to offer industrial AI solutions that work for the majority of cases, and that can leverage cross-domain knowledge to become better at solving new and more challenging problems over time.
What do you see as the most promising/interesting recent developments in deep learning, and how might they be applicable to industry?
The most promising development is certainly that of Graph Neural Networks (GNN) which are a type of deep learning model that can natively handle data that is not arranged on a grid, as is the case with conventional images, text, and videos. These methods are changing how computer vision is done, and enable faster and more successful drug discovery for example (see our recent paper on this), just to cite a few examples. In industrial AI, data is collected asynchronously from various sensors, with known spatial arrangement and dependencies, i.e. a sensor graph. A GNN can work on this data and leverage the structure of the domain as a strong regularizer to better generalize, for instance, in low data regime conditions.
The other area that is very relevant to achieving scalable industrial AI solutions is meta-learning, or learning to learn. Think of deploying a model in a factory and to being able to successfully transfer it to a (slightly) different factory almost seamlessly, without any further heavy lifting from your R&D team. This is also related to the emerging field of Offline RL, which we will cover in a future edition of our NNewsletter.
What are the differences between an AI project and a conventional engineering project?
A lot of what we do in AI involves engineering, of course. A good model gives you only a part, albeit essential, of the solution. However, the biggest difference with conventional engineering projects is that the trajectory to follow is often not clearly written a priori and must be discovered through an iterative process. Working with an experienced partner like NNAISENSE helps to mitigate this risk, to navigate close to optimal development. That being said, the industry also needs to go through a paradigm shift in how to approach AI projects, similar to what happened with agile software development. And last but not least, breaking with established patterns takes courage. If you want to get ahead of the competition, you need to be an early adopter of AI, and walk the rough path to success for better, more efficient and safer industrial processes.
AI Industry News
IoT, Industry 4.0, manufacturing, industrial processes
Key Takeaways from The Global AI Talent Tracker report
• The United States has a large lead over all other countries in top-tier AI research, with nearly 60% of top-tier researchers working for American universities and companies. The US lead is built on attracting international talent, with more than two-thirds of the top-tier AI researchers working in the United States having received undergraduate degrees in other countries.
• China is the largest source of top-tier researchers, with 29% of these researchers having received undergraduate degrees in China. But the majority of those Chinese researchers (56%) go on to study, work, and live in the United States.
• Over half (53%) of all the top-tier AI researchers are immigrants or foreign nationals currently working in a different country from where they received their undergraduate degrees.
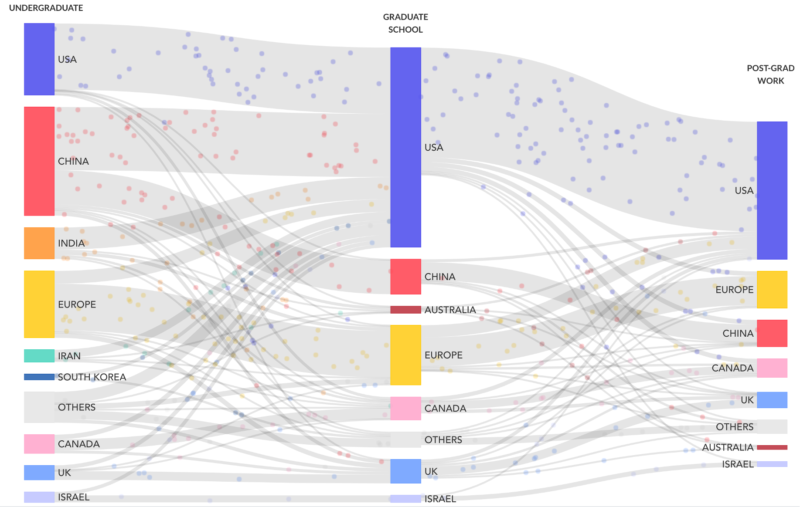
And where is Europe?
While Europe produces AI undergraduates at a level comparable to that of the US, when it comes to place of work, the US is the more likely destination by a factor of 4-5 times. And, Europeans who do their graduate work in the US tend to stay in the US to work. In short, we see a huge brain drain away from Europe to the US. Do we not value our own AI talent?
In order to reverse this development, Europe must do more than draft policies and dramatically increase investment into AI business opportunities such as Industrial AI in order to both retain homegrown talent and make Europe attractive to researchers from around the world.
All data is from a sample of authors of papers accepted at NeurIPS 2019. Country affiliations are based on the geographic location of the researcher, not their institution’s headquarters. For “Graduate School” column, all current and former graduate students are included. For “Post-Grad Work” column, only researchers who have completed their graduate studies are included. Creating this chart required excluding a small number of researchers for whom complete data was not available. Where there are differences in the percentages listed here from other statistics in the report, that exclusion is the reason.
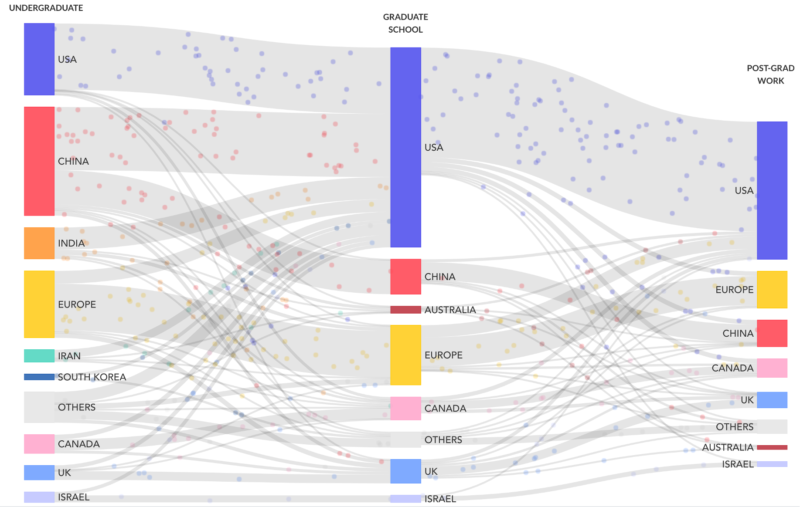
The State of AI Report 2020
Now in its third year, the State of AI Report 2020 analyses the most interesting developments in AI and features invited contributions from a range of well-known and up-and-coming companies and research groups. The Report considers the following key dimensions:
• Research: Technology breakthroughs and capabilities.
• Talent: Supply, demand and concentration of AI talent.
• Industry: Areas of commercial application for AI and its business impact.
• Politics: Regulation of AI, its economic implications and the emerging geopolitics of AI.
• Predictions: What we believe will happen and a performance review to keep us honest.
See report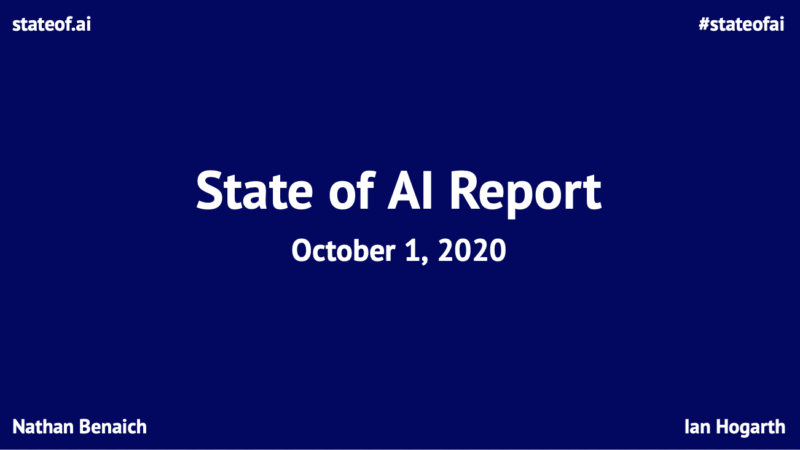
Nnaisense News
New patent granted
On April 20, the US Patent and Trademark Office will issue NNAISENSE patent #10984320 for Highly Trainable Neural Network Configuration based on Training Very Deep Networks which introduced the first trainable neural networks with hundreds of layers.
Congratulations to inventors Rupesh Srivastava (Senior Researcher at NNAISENSE) and Klaus Greff. This reflects our teams’ deep expertise in modern neural architectures that are driving progress in AI.
See Patent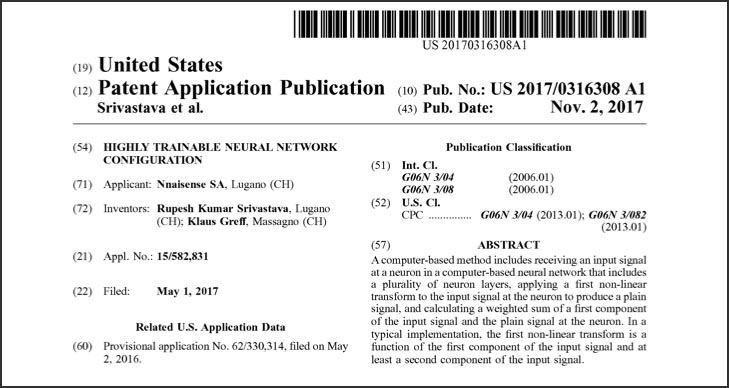
Switzerland Global Enterprise Report
Switzerland Global Enterprise, the Swiss government economic promotion agency placed NNAISENSE in their AI hot companies and ecosystem report.
We are gratified to receive Swiss recognition as a leading AI company after previously being listed in the DACH 30 and CB Insights AI 100.
“Often you are not seen as who you are in your very own company or country, while others have already given you recognition. We are of course honored to finally be in this list”
Ralf Haller, EVP Sales & Marketing, NNAISENSE
Read Swiss AI ecosystem report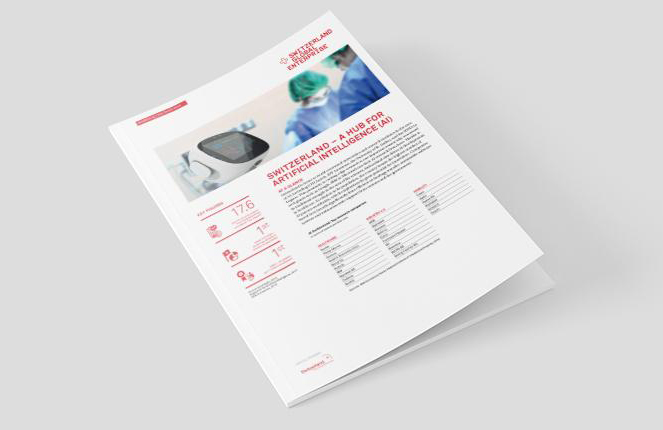
International Industrial AI Applications Summit
On March 24-26, NNAISENSE hosted this world-class (virtual) event, sponsored by Microsoft, with speakers from North America, China, Japan and Europe, and over 400 attendees.
You can watch the recorded presentations and panel discussions by simply registering via our website.
Enjoy speakers from Foxconn Technology (world’s largest industrial manufacturer with 80’000 robots installed), Prof. Jay Lee (who invented “Industrial AI”), NVIDIA, Intel, Microsoft, Continental, EOS, Sulzer & Schmid, SambaNova Systems, DFKI (German AI research center), Yokogawa, and our own experts presenting many of NNAISENSE’s installed use cases.
View Presentations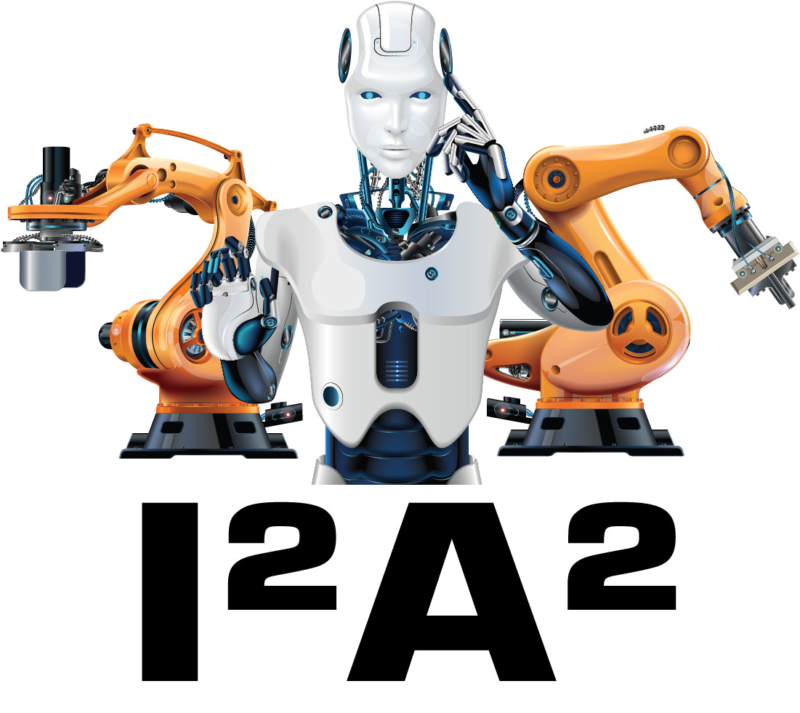
Windpower Data and Digital Innovation Forum
NNAISENSE Co-Founder, Jonathan Masci, presented “Taking rotor blades O&M to the next level in the age of digitalization” along with Tom Sulzer, CEO of our partner Sulzer & Schmid Laboratories.
Forum Information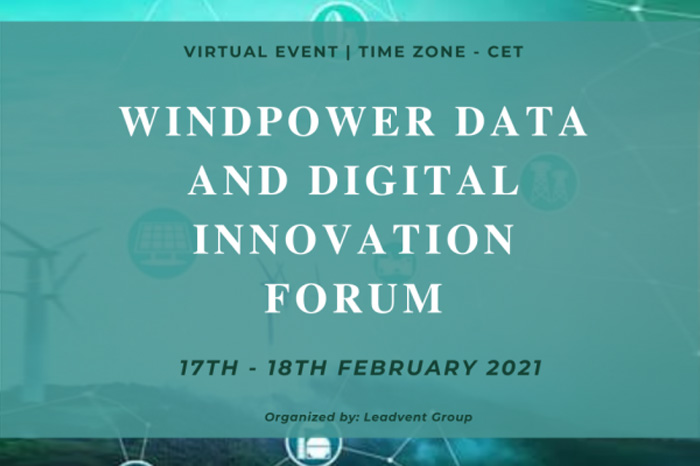
Recent Press
- Metrology.News
TRUMPF Partners with NNAISENSE Cutting – Edge AI Technology
(March 16, 2021)
- DevOps.com
How AI Will Drive Digital Twin 3.0
(March 15, 2021)
- Enterprise AI
Digital Twins and AI Helping Industries Stay Flexible During COVID-19 Uncertainties
(March 2, 2021) – Datanami Reprint
- Datanami
Digital Twins and AI Keep Industry Flexible During COVID-19
(March 1, 2021)
- Forbes
Can Digital Twins Change Our World? – quote from Faustino Gomez, Co-founder and CEO NNAISENSE
(February 11, 2021)